type
status
date
slug
summary
tags
category
icon
password
MiniMax has quietly progressed for two and a half years since its founding. At the recent MiniMax Link Partner Day, Yan Junjie, the founder, detailed the company's advancements in developing various multimodal models, including those for speech, music, and video generation. These models have shown leading technical prowess in multiple fields.
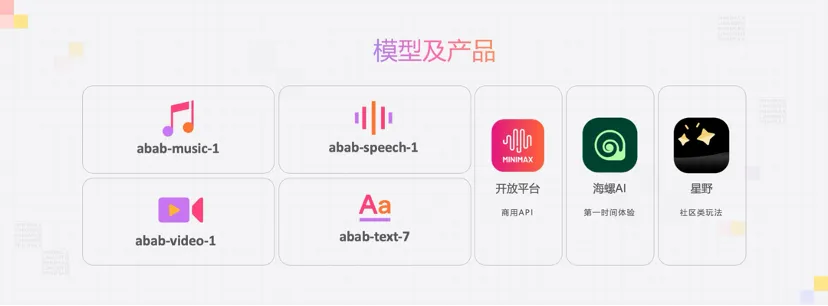
Notably, MiniMax has introduced its own AI video tool based on the DIT architecture: Conch Video.
This model, codenamed abab-video-1, excels in processing highly dynamic and ever-changing video content while maintaining a high compression rate.
It can simulate the physical laws of the real world and is adept at generating complex and high-action scenes.
It supports a variety of video styles, including 3D movie scenes, 2D animations, Chinese style, science fiction, and American comic styles, all of which can be easily controlled.
Additionally, it supports 3D text generation...
The abab-video-1 model has been evaluated using V-Bench, the "evaluation framework for video generation models," and it has achieved the top score, surpassing Keling and Runway.
Abab-video-1 boasts a high compression rate, excellent text responsiveness, diverse styles, and support for native high-resolution and high-frame-rate videos, providing a texture comparable to movies.

Introduction of models by MiniMax:
1. Voice Model
MiniMax's voice model has been meticulously refined and boasts numerous advanced features:
- Multi-language support: This model supports over 10 languages, including Japanese, Korean, Spanish, French, and Cantonese. MiniMax is the first company globally to offer a genuine Cantonese voice model.
- Emotional expression: The generated sentences are not only natural and fluent but also capable of simulating subtle emotional changes, making the voice expression more human-like and closely aligned with natural language expression.
- Music generation: The voice model also possesses the ability to generate music, creating highly artistic and versatile musical compositions, offering creators and users new experiences and surprises.

2. Music Model
MiniMax has introduced its first music generation model, celebrated for its artistic and flexible capabilities. Key features include:
- Highly anthropomorphic music generation: This model crafts intricate and emotional musical compositions, making it ideal for various creative scenarios and offering significant flexibility and innovation in music creation.
- Multi-style support: The model adeptly handles a wide range of music styles—from traditional instruments to modern electronic music, and from Chinese classical to Western pop.
3. Video Generation Model
MiniMax's video generation model ranks among the top video generation technologies globally, offering several distinct advantages:
- Strong text responsiveness: Leveraging MiniMax's extensive expertise in text processing, the model can precisely interpret and execute text instructions, creating video content that aligns closely with the given directives.
- High compression rate and dynamic expression: With MiniMax's proficiency in network architecture, the model excels in handling dynamic and complex video information while maintaining an efficient compression rate. This capability ensures the production of high-quality videos, particularly in intricate and high-action scenes.
- Style diversity: The model supports a wide range of video styles, including 3D movie scenes, 2D animations, Chinese style, science fiction, and American comic styles, all of which can be easily managed.
MiniMax has integrated these models into its open platform and related applications, such as the Xingye APP and Conch AI, allowing users to experience the latest models firsthand.
Next-Generation MOE+ Linear Attention Model by MiniMax
MiniMax has unveiled its new MOE+ Linear Attention-based model: abab 7, which rivals GPT-4o in performance.
abab 7 supports efficient training of vast datasets, significantly enhancing practicality and response speed while drastically reducing training and reasoning costs for large models. Compared to the traditional Transformer architecture, this new architecture cuts costs by over 90% at a sequence length of 128K, with even greater advantages as the sequence length increases.

The multimodal model abab 7, utilizing MoE+Linear Attention technology, will launch in a few weeks. Compared to the same generation model, GPT-4o, the new abab model has doubled its efficiency in processing 100,000 tokens, with even more notable improvements as the length increases.
Taking GPT-4o, Claude3.5 sonnet, and abab 7 as examples, it's evident that as input lengthens, the speed improvement is significantly greater compared to non-Linear Attention models. When processing 100,000 tokens, the new model's efficiency can reach 2-3 times that of previous models, and the efficiency continues to increase substantially with longer lengths. In theory, the model can process tokens of nearly infinite length.

- MOE (Mixture of Experts) architecture: The abab 7 model is built on MiniMax's proprietary MOE technology, which significantly boosts processing speed without compromising performance. This architecture allows the model to selectively activate certain experts, conserving computing resources while maintaining high efficiency and accuracy in specific tasks.
- Linear Attention: Abab 7 incorporates MiniMax's groundbreaking Linear Attention mechanism, enabling the model to handle extremely long input sequences with linear complexity. This enhancement not only improves performance in long text processing but also reduces error rates in complex tasks.
- Multimodal understanding and generation: Abab 7 excels in text generation and boasts robust multimodal processing capabilities. It can process and generate content across various forms, including images, sounds, and videos. Particularly in speech and video generation, abab 7 demonstrates a deep understanding of multimodal input, producing highly realistic and varied content.
Performance and Uses of Models by MiniMax
- Processing Speed and Efficiency: The combination of MOE and Linear Attention has enhanced abab 7's processing speed by several orders of magnitude compared to previous models. It excels in processing long sequences and executing complex tasks, achieving processing efficiency several times greater than that of traditional models.
- Generation Quality: In text generation, speech synthesis, or video creation, abab 7 has demonstrated exceptional generation quality. Its output is not only natural and fluent but also achieves remarkable accuracy in emotional expression and detail processing, nearly matching human creative ability.
- Multi-language and Multi-modal Support: Abab 7 handles multiple languages, including translation and emotional speech synthesis. It also supports generating multi-modal content, such as images and videos from text, offering users diverse and creative AI application scenarios.
Background Overview
Linear Attention optimizes the attention mechanism in the Transformer model, addressing the rapid increase in computational complexity as input lengthens. In traditional Transformers, this complexity is quadratic with input length (O(n^2)), making calculations costly and challenging for large inputs. Linear Attention seeks to lower this complexity to a linear relationship (O(n)), greatly enhancing model processing efficiency, particularly for long texts or large-scale data inputs.
How It Works
The core idea of Linear Attention is to simplify the traditional attention mechanism, thereby reducing computing resource consumption. This involves several key steps:
- Multiplication Approximation: In the traditional Transformer, the attention mechanism involves left and right multiplication, forming a dense matrix calculation. Linear Attention breaks this into two steps: first the left multiplication, followed by the right. By finding a suitable approximation method, computational complexity is effectively reduced.
- Normalization Replacement: The traditional Transformer uses the Softmax normalization function, which is computationally intensive. Linear Attention introduces a new normalization method that replaces Softmax while maintaining high efficiency in large-scale models.
- Position Encoding Optimization: Position encoding is crucial for preserving sequence order in the Transformer model. Linear Attention optimizes this to better adapt to linear calculations, further reducing computational complexity while maintaining model performance.
Technical Advantages
Linear Attention offers several significant benefits:
- Linear Computational Complexity: Unlike the traditional attention mechanism's O(n^2) complexity, Linear Attention reduces it to O(n), ensuring the model's efficiency when handling very long sequences.
- Efficient Long Sequence Processing: With linear computational complexity, Linear Attention can manage extremely long input sequences (e.g., over 100,000 tokens) without encountering resource bottlenecks.
- Improved Resource Utilization: The enhanced computing efficiency of Linear Attention allows for greater data processing under the same resource conditions. This accelerates the model's training and reasoning processes, which is particularly crucial for training large models.
MiniMax has effectively implemented Linear Attention technology in its latest model, applying it to large-scale model training and reasoning. The MiniMax team achieved this through innovative normalization methods and position encoding technology, successfully developing a new generation of models that can rival the world's top models, such as GPT-4.
In performance tests, the model using Linear Attention achieves 2-3 times the processing efficiency of non-Linear Attention models when handling 100,000-token inputs, with even greater efficiency improvements as input length increases. This capability allows the MiniMax model to excel in long text generation and complex task processing, significantly reducing error rates in large-scale, multi-step complex tasks.
MiniMax also announced that its large model interacts with users worldwide 3 billion times daily, including:
- Over 3 trillion text tokens processed daily, equivalent to experiencing 3,000 life experiences in one day.
- An average of 20 million images generated daily, akin to the painting collections of 400 Forbidden City palaces.
- An average of 70,000 hours of speech synthesis per day, comparable to reading 7,000 books a day.
Use cases:
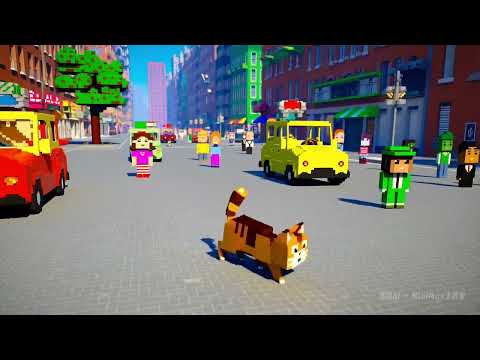
Experience it here: https://hailuoai.com/
- Author:KCGOD
- URL:https://kcgod.com/minimax-launches-voice-music-video-llm
- Copyright:All articles in this blog, except for special statements, adopt BY-NC-SA agreement. Please indicate the source!
Relate Posts
Google Launches Gemini-Powered Vids App for AI Video Creation
FLUX 1.1 Pro Ultra: Revolutionary AI Image Generator with 4MP Resolution
X-Portrait 2: ByteDance's Revolutionary AI Animation Tool for Cross-Style Expression Transfer
8 Best AI Video Generators Your YouTube Channel Needs
Meta AI’s Orion AR Glasses: Smart AI-Driven Tech to Replace Smartphones